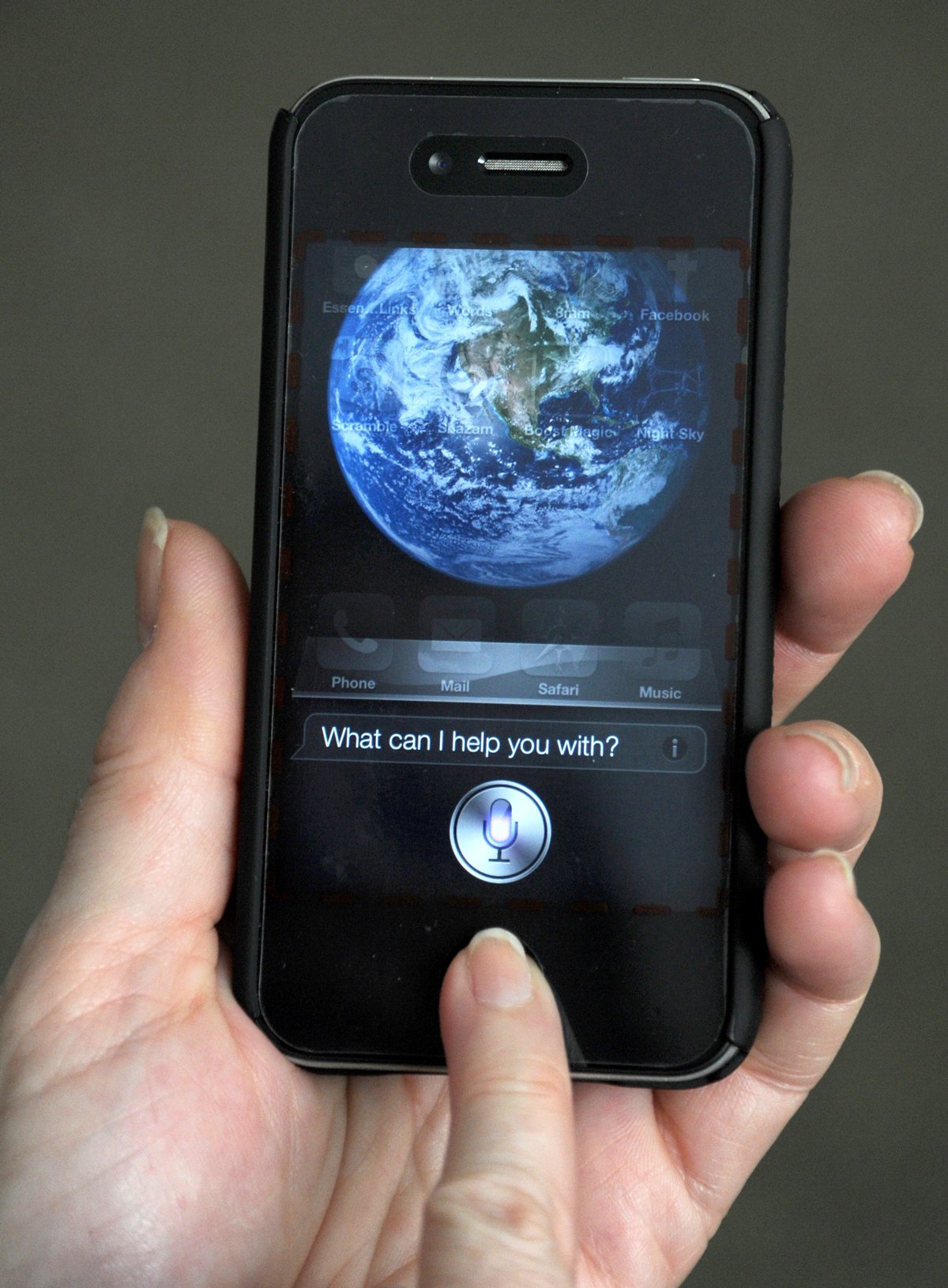
Try holding even a short conversation with Siri, Cortana, or Alexa and you may end up banging your head against the nearest wall in frustration.
Voice assistants are often good at responding to simple queries, but they struggle with complicated requests or any sort of back-and-forth. This could start to change, however, as new machine-learning techniques are applied to the challenge of human-machine dialogue in the next few years.
Speaking at a major AI conference last week, Steve Young, a professor at the University of Cambridge who also works part time on Apple’s Siri team, talked about how recent advances are starting to improve dialogue systems. Young did not comment on his work at Apple but described his academic research.
Early voice assistants, including Siri, used machine learning for voice recognition but responded to language according to hard-coded rules. This is increasingly changing as machine-learning techniques are applied to parsing language (see “AI’s Language Problem”).
Young said in particular that reinforcement learning, the technique DeepMind used to build a program capable of beating one of the world’s best Go players, could help advance the state of the art significantly. Whereas AlphaGo learned by playing thousands of games against itself, and received positive reinforcement with each win, conversational agents could vary their responses and receive positive (or negative) feedback in the form of users’ actions.
“I think it’s got to be a big thing,” Young said of reinforcement learning when I spoke to him after his talk. “The most powerful asset you have is the user.”
Young said that voice assistants wouldn’t need to vary their behavior dramatically for this to have an effect. They might simply try performing an action in a slightly different way. “You can do it in a very controlled way,” he said. “You don’t have to do daft things.”
During his talk, Young explained why parsing language is so difficult for machines. Unlike image recognition, for example, language is compositional, meaning the same components can be rearranged to produce vastly different meanings. Another key challenge with language is that it offers only an incomplete glimpse of what another person is thinking, so it is often necessary to make guesses about what a phrase or sentence means. On a practical level, as a spoken query gets longer, interpreting it often requires merging knowledge from different domains. For instance, a complex query about a restaurant may require an understanding of time, location, and food.
Still, Young believes that the time is right for conversational assistants to get a whole lot better. “The commercial demand is there, and the technology is there,” he says. “I think over the next five years you will see really significant progress.”
Young joined Apple after the company acquired his startup, VocalIQ, in 2015. Apple has been accused of falling behind competitors in the race to exploit technology based on advances in machine learning and AI, but Young’s work suggests that this is far from true. And the company has also been making efforts to open up its AI research in order to attract top talent. The company recently hired Ruslan Salakhutdinov, a professor from Carnegie Mellon University, to serve as its first director of AI, and its researchers have begun presenting and publishing papers for the first time (see “Apple Gets Its First Director of AI”).
Apple isn’t the only company interested in conversational technology, of course. Amazon’s Alexa—a device for the home that relies entirely on voice control—has become a hit, and other companies have rushed to develop similar home helpers. Google’s offering, called Google Home, uses particularly advanced language-parsing techniques (see “Google’s Assistant Is More Ambitious Than Siri and Alexa”).
Researchers at IBM, in collaboration with a team from the University of Michigan, are also experimenting with conversational systems that exploit reinforcement learning. Satinder Baveja, a professor at the University of Michigan who is involved with that project, says reinforcement learning offers a powerful new way to train dialogue systems, but he doesn’t think Siri attain truly human-like communication skills in his lifetime.
“These systems will begin to use richer context,” he says. “Although I do think that they will remain limited in scope, addressing specific tasks like restaurant reservations, travel, tech support, and so on.”